What Is So Fascinating About Marijuana News?
The Meaning of Marijuana News
If you’re against using Cannabis as you do not need to smoke you’re misinformed. As there is barely any cannabis left in a roach, some people today argue that the song is all about running out of cannabis and not having the ability to acquire high, exactly like the roach isn’t able to walk because it’s missing a leg. If you’re thinking about consuming cannabis please consult your health care provider first. Before visiting test.com the list, it’s important to be aware of the scientific reason cannabis works as a medication generally, and more specifically, the scientific reason it can send cancer into remission. At the moment, Medical Cannabis was still being used to take care of several health-related problems. In modern society, it is just starting to receive the recognition it deserves when it comes to treating diseases such as Epilepsy.
In nearly all the nation, at the present time, marijuana is illegal. To comprehend what marijuana does to the brain first you’ve got to know the key chemicals in marijuana and the various strains. If you are a person who uses marijuana socially at the occasional party, then you likely do not have that much to be concerned about. If you’re a user of medicinal marijuana, your smartphone is possibly the very first place you start looking for your community dispensary or a health care provider. As an issue of fact, there are just a few types of marijuana that are psychoactive. Medical marijuana has entered the fast-lane and now in case you reside in Arizona you can purchase your weed without leaving your vehicle. Medical marijuana has numerous therapeutic effects which will need to be dealt with and not only the so-called addictive qualities.
If you’re using marijuana for recreational purposes begin with a strain with a minimal dose of THC and see the way your body reacts. Marijuana is simpler to understand because it is both criminalized and decriminalized, based on the place you go in the nation. If a person is afflicted by chronic depression marijuana can directly affect the Amygdala that is accountable for your emotions.
Much enjoy the wine industry was just two or three decades past, the cannabis business has an image problem that’s keeping people away. In the event you want to learn where you are able to find marijuana wholesale companies near you, the very best place to seek out such companies is our site, Weed Finder. With the cannabis industry growing exponentially, and as more states start to legalize, individuals are beginning to learn that there is far more to cannabis than simply a plant that you smoke. In different states, the work of legal marijuana has produced a patchwork of banking and tax practices. Then the marijuana sector is ideal for you.
Marijuana News for Dummies
Know what medical cannabis options can be found in your state and the way they respond to your qualifying medical condition. They can provide medicinal benefits, psychotropic benefits, and any combination of both, and being able to articulate what your daily responsibilities are may help you and your physician make informed, responsible decisions regarding the options that are appropriate for you, thus protecting your employment, your family and yourself from untoward events. In the modern society, using drugs has become so prevalent it has come to be a component of normal life, irrespective of age or gender. Using marijuana in the USA is growing at a quick rate.
How Restaurants Can Effectively Use Chatbots?
Creating an engaging and intuitive chatbot experience is crucial for ensuring user satisfaction and effectiveness. Follow this step-by-step guide to design a chatbot that meets your restaurant’s needs and delights your customers. To do so, drag a green arrow from the green corresponding to the “Show me the menu! ” button and when a features menu appears, select the “SET VARIABLE” block.
Chatbots for restaurants, like ChatBot, are essential in improving the ordering and booking process. Customers can easily communicate their preferences, dietary requirements, and preferred reservation times through an easy-to-use conversational interface. Serving as a virtual assistant, the chatbot ensures customers have a seamless and tailored experience. Restaurants may maximize their Chat PG operational efficiency and improve customer happiness by utilizing this technology. AI-powered conversational interfaces provide numerous benefits for restaurants compared to traditional channels like phone calls and paper menus. As the technology behind natural language processing and chatbots continues advancing, we can expect them to become more seamless, personalized and ubiquitous.
We don’t support Messenger chatbots so if you are trying to engage customers on that platform, we aren’t the builder for you. Restaurant chatbots are like helpful computer programs for restaurants. They can do things such as taking reservations, showing menus to customers, and even taking orders. From automating reservations and answering customer inquiries to boosting online orders and improving overall dining experiences chatbots can do it all.
They are also cost-effective and can chat with multiple people simultaneously. You can use a chatbot restaurant reservation system to make sure the bookings and orders are accurate. You can also deploy bots on your website, app, social media accounts, or phone system to interact with customers quickly.
Domino’s Pizza Chatbot
Restaurant chatbots are designed to automate specific responsibilities carried out by human staff, like booking reservations. Chatbots might have a variety of skills depending on the use case they are deployed for. TGI Fridays use a restaurant bot to serve a variety of customer needs. These include placing an order, finding the nearest restaurant, and contacting the business.
Conversational AI and chatbots have exploded in popularity across industries, especially in the restaurant space. Once the query of the customer is resolved it makes sense to end the conversation. When users push the end of the chat button they can direct a very short survey regarding their experience with chatbot.
- According to Hospitality Technology, up to 30% of online reservations are no-shows when there are no confirmations.
- This proactive approach helps maintain high ratings for your restaurant’s quality service.
- In this section, we’ll discuss some key things to remember when creating a restaurant chatbot.
- Second, Messenger (and Kik and Telegram) bots all face a discovery issue.
Sometimes all you need is a little bit of inspiration and real-life examples, not just dry theory. When you click on the next icon, you’ll be able to personalize the cards on the decision card messages. You can change the titles, descriptions, images, and buttons of your cards. These will all depend on your restaurant and what are your frequently asked questions. Fill the cards with your photos and the common choices for each of them.
Implement Conversational Voice Interfaces
It’s important for restaurants to have their own chatbot to be able to talk to customers anytime and anywhere. The bot can be used for customer service automation, making reservations, and showing the menu with pricing. They can assist both your website visitors on your site and your Facebook followers on the platform.
Wendy’s to test AI chatbot at Ohio drive-thru – The Hill
Wendy’s to test AI chatbot at Ohio drive-thru.
Posted: Wed, 10 May 2023 07:00:00 GMT [source]
Using an app feels like using a tool to achieve something, while using a bot feels like the computer is assisting you through a process. Second, if you build a bot within a messaging app like FB Messenger, you can trust Facebook’s highly paid and highly trained UI team to make the interface responsive. Second, if you are willing to sacrifice the complexity of the interaction, you https://chat.openai.com/ do not need AI to create a good and cheap conversational commerce experience. Furthermore, Panda Express provides a platform for clients to submit suggestions and complaints through the bot to swiftly gather customer feedback. This innovative system offers customers a convenient and efficient way to order pizza, significantly reducing the load on the website and mobile app.
Restaurant bots can also perform tedious tasks and minimize human error in bookings and orders. By automating these tasks, chatbots can help save time and improve efficiency for restaurant staff. This, in turn, can lead to a more promising overall customer experience. Chatbots can be integrated with a restaurant’s ordering system to allow customers to place orders via messaging platforms or the restaurant’s website. Integrating a chatbot with your website or mobile app is a walk in the park. With the rise of voice search, enable customers to place orders, make reservations, and interact with your bot using natural speech.
A restaurant chatbot is an excellent tool for providing concierge services to your customers. Restaurant chatbots can assist customers in enrolling and registering, for the loyalty program directly through the chat interface ensuring a smooth registration experience. By integrating with the loyalty program database, bots provide customers with up-to-date information on their accumulated points, giving a clear understanding of their potential rewards. Whether customers are eating in your restaurant or ordering for takeaway, a restaurant reservation chatbot is there to assist them. The bot’s user-friendly interface can provide customers with an itemized menu that they can easily navigate to place orders. Customer feedback is critical to the success of any restaurant, and a chatbot can be a great help here.
Second, I would try and figure out which platform you want to build your bot on. Facebook Messenger is fairly universally used so bot developers tend to gravitate towards it. But if you are in a region where another messaging app is popular then build a bot on that platform (Line, Kik, Telegram, etc).
Unlike generalized virtual assistants, restaurant chatbots are highly customized for industry-specific features like taking food orders, answering menu questions, and reservations. Restaurant chatbots provide businesses an edge in a time when fast, tailored, and efficient customer service is important. Using chatbots in restaurants is not a fad but a strategic move to boost efficiency, customer satisfaction, and company success as technology progresses.
In addition to text, have your chatbot send images of menu items, restaurant ambiance, prepared dishes, etc. Visuals make conversations more engaging while showcasing offerings. According to Hospitality Technology, up to 30% of online reservations are no-shows when there are no confirmations. Restaurant chatbots can help reduce no-shows by automatically sending reservation confirmations and reminders. Before finalizing the chatbot, conduct thorough testing with real users to identify any issues or bottlenecks in the conversation flow.
From reservations to waitlist updates, let AI Chatbot simplify operations, ensuring a seamless and delightful dining journey. By offering packages at a discounted price, bots can increase the overall value proposition for customers and drive revenue growth for your restaurant. The restaurant bot can also display daily offers and answer queries- all without any human assistance. 33% of consumers want to be able to use a chatbot to make a reservation at a hotel or restaurant. Code it yourself, or use one of the many chatbot building platforms that allow you to do so without code. When a customer interacts with a bot and an app the two experiences feel very different even if they achieve the same thing.
Chatbots, like our own ChatBot, are particularly good at responding swiftly and accurately to consumer questions. This skill raises customer happiness while also making a big difference in the overall effectiveness of restaurant operations. Creating a seamless dining experience is the ultimate goal of chatbots used in restaurants. Chatbots are crucial in generating a great and memorable client experience by giving fast and accurate information, making transactions simple, and making tailored recommendations.
Restaurant chatbots can also recognize returning customers and use previous purchase information to advise the visitor. A bot can suggest dishes a customer may not know about, or recommend the best drink to match their preferred meal. You can use them to manage orders, increase sales, answer frequently asked questions, and much more. A chatbot is used by the massive international pizza delivery company Domino’s Pizza to expedite the ordering process. Through the chatbot interface, customers can track delivery, place orders, and receive personalized recommendations, enhancing the convenience of the overall experience.
You can easily download and customize our ready-to-use restaurant chatbot template or create your own from scratch. By following these best practices and using Tiledesk’s chatbot template, you can create a chatbot that is effective, engaging, and easy to use for both your customers and your staff. Next, designing a chatbot that fits your restaurant’s brand and voice is important. A well-designed chatbot can help build customer trust and loyalty, so consider the tone and style of your chatbot’s responses.
By integrating chatbots in this way, restaurants can remain dynamic and flexible, constantly changing to meet the needs of their clients. This means that guests can have their inquiries and concerns addressed immediately, regardless of the time of day or night. Let them avail of various restaurant services at their convenience. Offering chatbot for restaurant 24/7 support through our restaurant bot helps you stand out from your competitors and attract customers who value accessibility and convenience. The three most prominent users of chatbots in the restaurant space are Domino’s, TGI Friday and Pizza Hut. Dominos and Pizzahut use it for food ordering and TGI Friday for making reservations.
How to build a restaurant chatbot
In the next few sections, we show you the advantages of deploying a Conversational AI chatbot in your restaurant or food delivery business. Data shows customers are 67% more likely to book tables using a restaurant‘s chatbot compared to calling. For the sake of this tutorial, we will use Tidio to customize one of the templates and create your first chatbot for a restaurant.
In the long run, this can build trust in your website, delight clients, and gain customer loyalty to your restaurant. Chippy uses artificial intelligence to replicate Chipotle’s exact chip-making recipe, which results in frying chips to perfection, the company said. Tech companies such as ConverseNow are swiftly reshaping how restaurant chains including Domino’s and Wingstop take phone orders. A June Deloitte consumer survey found that consumers were also more willing to frequent restaurants that used automation.
They can also be transferred to your support agents by typing a question. You can change the last action to a subscription form, customer satisfaction survey, and more. Because chatbots are direct lines of communication, restaurants may easily include them in their marketing campaigns. Customers feel more connected and loyal as a result of this open channel of communication, which also increases the efficacy of marketing activities. The fast-casual fresh-Mex chain from Newport Beach, California, was an early adopter of voice bots.
UKB199 also provides a diverse array of questions to choose from, covering aspects like restaurant location, contact number, pricing, and reservation options. This feature enables customers to effortlessly place orders and make payments for their food and beverages through voice commands. Furthermore, it allows for on-the-fly modifications to their drink orders, mimicking a real-life conversation with a barista. I am Paul Christiano, a fervent explorer at the intersection of artificial intelligence, machine learning, and their broader implications for society.
Renowned as a leading figure in AI safety research, my passion lies in ensuring that the exponential powers of AI are harnessed for the greater good. Throughout my career, I’ve grappled with the challenges of aligning machine learning systems with human ethics and values. My work is driven by a belief that as AI becomes an even more integral part of our world, it’s imperative to build systems that are transparent, trustworthy, and beneficial.
Restaurants, in particular, are influenced by customer feedback on platforms like Yelp and TripAdvisor. There is a way to make this happen and it’s called the “Persistent Menu” block. In essence, the block creates permanent buttons in the header of your chatbot. The restaurant industry has been traditionally slow to adopt new technology to attract customers.
It’s a win-win for everyone – customers get the information they need quickly, and your staff can focus on what they do best. The restaurant reservation bots can suggest complementary products or services to customers while placing orders, such as a dessert with a meal or a cold drink with a burger meal for two. If you do not know how to code don’t worry, because the internet has you covered. There are a lot of bot builders that let you create detailed conversational experiences with no coding experience whatsoever. There are two things to consider before you start building your bot. First, I would think long and hard about what function your bot will serve.
Though the initial menu setup might take some time, remember you are building a brick which can be saved to your library as a reusable block. Formulas block allows you to make all kinds of calculations and processes similar to those you can do in Excel or Google Spreadsheets inside the Landbot builder. Drag an arrow from the menu item you want to “add to cart” and select “Formulas” block from the features menu.
You can foun additiona information about ai customer service and artificial intelligence and NLP. Customizing this block is a great way to familiarize yourself with the Landbot builder. As you can see, the building of the chatbot flow happens in the form of blocks. Each block represents one turn of the conversation with the text/question/media shared by the chatbot followed by the user answer in the form of a button, picture, or free input.
The examples we gave above of the AI fail and the hotel booking were both examples of chatbots. In the sections 1 and 2, I am going to explain what conversational commerce is and why there is growing buzz around it in the tech space. In section 3, I will discuss what this new tech trend means for the restaurant industry in particular. Finally, section 4 will give you resources you need to get started. It’s essential to offer users the option to end a chat once their query is resolved. This practice allows for the collection of valuable feedback through brief surveys regarding the chatbot’s performance.
McDonald’s taps Google for ‘Ask Pickles’ AI chatbot to help fix ice cream machines – Yahoo Finance
McDonald’s taps Google for ‘Ask Pickles’ AI chatbot to help fix ice cream machines.
Posted: Thu, 07 Dec 2023 08:00:00 GMT [source]
Despite their benefits, many chain restaurant owners and managers are unaware of restaurant chatbots. This article aims to close the information gap by providing use cases, case studies and best practices regarding chatbots for restaurants. This restaurant chatbot asks four questions at the start, but they seem more human-like than the robotic options of “Menu”, “Opening hours”, etc. This makes the conversation a little more personal and the visitor might feel more understood by the business. You can choose from the options and get a quick reply, or wait for the chat agent to speak to.
Artificial Intelligence (AI) is slowly enabling us to shift back to a paradigm where the user does less on their own. An ideal AI travel assistant would be able to take your travel requirements and book all the flights and hotels you need in one bundle like a travel agent. Unlike a travel agent though, they could do it instantly like an app and for cheaper because there is no human that needs to be paid sitting at the back. Computers cease to be a tool used to do something yourself and more an assistant that is doing things for you. Till recently, the solution has been to get customers to serve themselves. If you have ever gone to a corner store, pharmacy or a shopping mall and talked to any of the store attendants you have engaged in conversational commerce.
This feature is especially important for global chains or small businesses that serve a wide range of customers with different schedules. In addition to quickly responding to consumer inquiries, the round-the-clock support option fosters client loyalty and trust by being dependable. Chatbots are useful for internal procedures and customer interactions. Chatbots for restaurants function as interactive interfaces for guests, enabling them to place orders, schedule appointments, and request information in a conversational way. A more personalized and engaging experience is made possible by focusing on natural language, which strengthens the bond between the visitor and the restaurant.
Chatbot restaurant reservations are artificial intelligence (AI) systems that make use of machine learning (ML) and natural language processing (NLP) techniques. Thanks to this technology, these virtual assistants can replicate human-like interactions by understanding user inquiries and responding intelligently. This pivotal element modifies the customer-service dynamic, augmenting the overall interaction.
Getting Started with Sentiment Analysis using Python
As the last step before we train our algorithms, we need to divide our data into training and testing sets. The training set will be used to train the algorithm while the test set will be used to evaluate the performance of the machine learning model. We need to clean our tweets before they can be used for training the machine learning model. However, before cleaning the tweets, let’s divide our dataset into feature and label sets.
After reviewing the tags, exit the Python session by entering exit(). Normalization helps group together words with the same meaning but different forms. Without normalization, “ran”, “runs”, and “running” would be treated as different words, even though you may want them to be treated as the same word.
Subsequently, the precision of opinion investigation generally relies upon the intricacy of the errand and the framework’s capacity to gain from a lot of information. And, because of this upgrade, when any company promotes their products on Facebook, they receive more specific reviews which will help them to enhance the customer experience. In a time overwhelmed by huge measures of computerized information, understanding popular assessment and feeling has become progressively pivotal. This acquaintance fills in as a preliminary with investigate the complexities of feeling examination, from its crucial ideas to its down to earth applications and execution.
Enough of the exploratory data analysis, our next step is to perform some preprocessing on the data and then convert the numeric data into text data as shown below. There are many sources of public sentiment e.g. public interviews, opinion polls, surveys, etc. However, with more and more people joining social media platforms, websites like Facebook and Twitter can be parsed for public sentiment.
So, very quickly, NLP is a sub-discipline of AI that helps machines understand and interpret the language of humans. It’s one of the ways to bridge the communication gap between man and machine. Basically, it describes the total occurrence of words within a document. For example, “run”, “running” and “runs” are all forms of the same lexeme, where the “run” is the lemma.
Feature/aspect-based
As we humans communicate with each other in a way that we call Natural Language which is easy for us to interpret but it’s much more complicated and messy if we really look into it. Now, as we said we will be creating a Sentiment Analysis using NLP Model, but it’s easier said than done. The second review is negative, and hence the company needs to look into their burger department. Sentiment Analysis, as the name suggests, it means to identify the view or emotion behind a situation. It basically means to analyze and find the emotion or intent behind a piece of text or speech or any mode of communication. One of, if not THE cleanest, well-thought-out tutorials I have seen!
- A comparison of stemming and lemmatization ultimately comes down to a trade off between speed and accuracy.
- See the
Natural Language API Reference for complete
information on the specific structure of such a request.
- Hence, we are converting all occurrences of the same lexeme to their respective lemma.
- Thanks for taking the time and going to the trouble to get it right.
And then, we can view all the models and their respective parameters, mean test score and rank as GridSearchCV stores all the results in the cv_results_ attribute. Scikit-Learn provides a neat way of performing the bag of words technique using CountVectorizer. But first, we will create an object of WordNetLemmatizer and then we will perform the transformation. Now, we will concatenate these two data frames, as we will be using cross-validation and we have a separate test dataset, so we don’t need a separate validation set of data. And, the third one doesn’t signify whether that customer is happy or not, and hence we can consider this as a neutral statement.
Sentiment analysis is a common NLP task, which involves classifying texts or parts of texts into a pre-defined sentiment. You will use the Natural Language Toolkit (NLTK), a commonly used NLP library in Python, to analyze textual data. In many social networking services or e-commerce websites, users can provide text review, comment or feedback to the items. These user-generated text provide a rich source of user’s sentiment opinions about numerous products and items.
We performed an analysis of public tweets regarding six US airlines and achieved an accuracy of around 75%. I would recommend you to try and use some other machine learning algorithm such as logistic regression, SVM, or KNN and see if you can get better results. These challenges highlight the complexity of human language and communication.
Download App
In our case, it took almost 10 minutes using a GPU and fine-tuning the model with 3,000 samples. The more samples you use for training your model, the more accurate it will be but training could be significantly slower. The problem is that most sentiment analysis algorithms use simple terms to express sentiment about a product or service. In the code above, we define that the max_features should be 2500, which means that it only uses the 2500 most frequently occurring words to create a “bag of words” feature vector.
Now you’ve reached over 73 percent accuracy before even adding a second feature! While this doesn’t mean that the MLPClassifier will continue to be the best one as you engineer new features, having additional classification algorithms at your disposal is clearly advantageous. Many of the classifiers that scikit-learn provides can be instantiated quickly since they have defaults that often work well. In this section, you’ll learn how to integrate them within NLTK to classify linguistic data. One of them is .vocab(), which is worth mentioning because it creates a frequency distribution for a given text.
One direction of work is focused on evaluating the helpfulness of each review.[76] Review or feedback poorly written is hardly helpful for recommender system. Besides, a review can be designed to hinder sales of a target product, thus be harmful to the recommender system even it is well written. Subsequently, the method described in a patent by Volcani and Fogel,[5] looked specifically at sentiment and identified individual words and phrases in text with respect to different emotional scales.
A current system based on their work, called EffectCheck, presents synonyms that can be used to increase or decrease the level of evoked emotion in each scale. Similarly, max_df specifies that only use those words that occur in a maximum of 80% of the documents. Words that occur in all documents https://chat.openai.com/ are too common and are not very useful for classification. Similarly, min-df is set to 7 which shows that include words that occur in at least 7 documents. From the output, you can see that the confidence level for negative tweets is higher compared to positive and neutral tweets.
However, training this model on 2 class data using higher dimension word vectors achieves the 87 score reported in the original CNN classifier paper. On a three class projection of the SST test data, the model trained on multiple datasets gets 70.0%. The Chat PG SentimentProcessor adds a label for sentiment to each Sentence. The existing models each support negative, neutral, and positive, represented by 0, 1, 2 respectively. Custom models could support any set of labels as long as you have training data.
If you would like to use your own dataset, you can gather tweets from a specific time period, user, or hashtag by using the Twitter API. This article assumes that you are familiar with the basics of Python (see our How To Code in Python 3 series), primarily the use of data structures, classes, and methods. The tutorial assumes that you have no background in NLP and nltk, although some knowledge on it is an added advantage. DocumentSentiment.score
indicates positive sentiment with a value greater than zero, and negative
sentiment with a value less than zero.
Sentiment analysis goes beyond that – it tries to figure out if an expression used, verbally or in text, is positive or negative, and so on. To get a relevant result, everything needs to be put in a context or perspective. When a human uses a string of commands to search on a smart speaker, for the AI running the smart speaker, it is not sufficient to “understand” the words. NLP is used to derive changeable inputs from the raw text for either visualization or as feedback to predictive models or other statistical methods. This post’s focus is NLP and its increasing use in what’s come to be known as NLP sentiment analytics. Now, we will check for custom input as well and let our model identify the sentiment of the input statement.
Hands-On House Price Prediction – Machine Learning in Python
Sentiment analysis can be used to categorize text into a variety of sentiments. For simplicity and availability of the training dataset, this tutorial helps you train your model in only two categories, positive and negative. Sentiment Analysis inspects the given text and identifies the prevailing
emotional opinion within the text, especially to determine a writer’s attitude
as positive, negative, or neutral.
- Similarly, max_df specifies that only use those words that occur in a maximum of 80% of the documents.
- It basically means to analyze and find the emotion or intent behind a piece of text or speech or any mode of communication.
- You’ll notice lots of little words like “of,” “a,” “the,” and similar.
- So how can we alter the logic, so you would only need to do all then training part only once – as it takes a lot of time and resources.
- The training set will be used to train the algorithm while the test set will be used to evaluate the performance of the machine learning model.
To solve this problem, we will follow the typical machine learning pipeline. We will then do exploratory data analysis to see if we can find any trends in the dataset. Next, we will perform text preprocessing to convert textual data to numeric data that can be used by a machine learning algorithm.
You can use any of these models to start analyzing new data right away by using the pipeline class as shown in previous sections of this post. You can foun additiona information about ai customer service and artificial intelligence and NLP. This section demonstrates a few ways to detect sentiment in a document. The above example would indicate a review that was relatively positive. (score of 0.5), and relatively emotional (magnitude of 5.5). Have a little fun tweaking is_positive() to see if you can increase the accuracy. Note that .concordance() already ignores case, allowing you to see the context of all case variants of a word in order of appearance.
Sentiment analysis is a branch of natural language processing (NLP) that involves using computational methods to determine and understand the sentiments or emotions expressed in a piece of text. The goal is to identify whether the text conveys a positive, negative, or neutral sentiment. Python offers several powerful packages for sentiment analysis and here is a concise overview of the top 5 packages.
It’s less accurate when rating longer, structured sentences, but it’s often a good launching point. This will create a frequency distribution object similar to a Python dictionary but with added features. While you’ll use corpora provided by NLTK for this tutorial, it’s possible to build your own text corpora from any source. Building a corpus can be as simple as loading some plain text or as complex as labeling and categorizing each sentence. Refer to NLTK’s documentation for more information on how to work with corpus readers. NLTK provides a number of functions that you can call with few or no arguments that will help you meaningfully analyze text before you even touch its machine learning capabilities.
AutoNLP will automatically fine-tune various pre-trained models with your data, take care of the hyperparameter tuning and find the best model for your use case. All models trained with AutoNLP are deployed and ready for production. Finally, to evaluate the performance of the machine learning models, we can use classification metrics such as a confusion matrix, F1 measure, accuracy, etc. Once you’re left with unique positive and negative words in each frequency distribution object, you can finally build sets from the most common words in each distribution. The amount of words in each set is something you could tweak in order to determine its effect on sentiment analysis. Further, they propose a new way of conducting marketing in libraries using social media mining and sentiment analysis.
All these classes have a number of utilities to give you information about all identified collocations. Another powerful feature of NLTK is its ability to quickly find collocations with simple function calls. Collocations are series of words that frequently appear together in a given text. In the State of the Union corpus, for example, you’d expect to find the words United and States appearing next to each other very often. You’ll notice lots of little words like “of,” “a,” “the,” and similar. These common words are called stop words, and they can have a negative effect on your analysis because they occur so often in the text.
With NLP, this form of analytics groups words into a defined form before extracting meaning from the text content. Sentiment analytics is emerging as a critical input in running a successful business. Want to know more about Express Analytics sentiment analysis service?
Many of NLTK’s utilities are helpful in preparing your data for more advanced analysis. Financial firms can divide consumer sentiment data to examine customers’ opinions about their experiences with a bank along with services and products. Both financial organizations and banks can collect and measure customer feedback regarding their financial products and brand value using AI-driven sentiment analysis systems. Now, we will read the test data and perform the same transformations we did on training data and finally evaluate the model on its predictions. We can make a multi-class classifier for Sentiment Analysis using NLP. But, for the sake of simplicity, we will merge these labels into two classes, i.e.
Each item in this list of features needs to be a tuple whose first item is the dictionary returned by extract_features and whose second item is the predefined category for the text. After initially training the classifier with some data that has already been categorized (such as the movie_reviews corpus), you’ll be able to classify new data. To further strengthen the model, you could considering adding more categories like excitement and anger. In this tutorial, you have only scratched the surface by building a rudimentary model.
Notice that the function removes all @ mentions, stop words, and converts the words to lowercase. The function lemmatize_sentence first gets the position tag of each token of a tweet. Within the if statement, if the tag starts with NN, the token is assigned as a noun. Similarly, if the tag starts with VB, the token is assigned as a verb.
The NLTK library contains various utilities that allow you to effectively manipulate and analyze linguistic data. Among its advanced features are text classifiers that you can use for many kinds of classification, including sentiment analysis. You will use the negative and positive tweets to train your model on sentiment analysis later in the tutorial. In this section, we’ll go over two approaches on how to fine-tune a model for sentiment analysis with your own data and criteria. The first approach uses the Trainer API from the 🤗Transformers, an open source library with 50K stars and 1K+ contributors and requires a bit more coding and experience.
(PDF) Fear of Artificial Intelligence? NLP, ML and LLMs Based Discovery of AI-Phobia and Fear Sentiment Propagation … – ResearchGate
(PDF) Fear of Artificial Intelligence? NLP, ML and LLMs Based Discovery of AI-Phobia and Fear Sentiment Propagation ….
Posted: Mon, 18 Mar 2024 09:04:56 GMT [source]
It is a data visualization technique used to depict text in such a way that, the more frequent words appear enlarged as compared to less frequent words. This gives us a little insight into, how the data looks after being processed through all the steps until now. Stopwords are commonly used words in a sentence such as “the”, “an”, “to” etc. which do not add much value. Sentiment analysis is a mind boggling task because of the innate vagueness of human language.
NLP Libraries
Language in its original form cannot be accurately processed by a machine, so you need to process the language to make it easier for the machine to understand. The first part of making sense of the data is through a process nlp sentiment called tokenization, or splitting strings into smaller parts called tokens. For training, you will be using the Trainer API, which is optimized for fine-tuning Transformers🤗 models such as DistilBERT, BERT and RoBERTa.
The second approach is a bit easier and more straightforward, it uses AutoNLP, a tool to automatically train, evaluate and deploy state-of-the-art NLP models without code or ML experience. Sentiment analysis allows processing data at scale and in real-time. For example, do you want to analyze thousands of tweets, product reviews or support tickets? Once data is split into training and test sets, machine learning algorithms can be used to learn from the training data. However, we will use the Random Forest algorithm, owing to its ability to act upon non-normalized data. Statistical algorithms use mathematics to train machine learning models.
Hence, we are converting all occurrences of the same lexeme to their respective lemma. Because, without converting to lowercase, it will cause an issue when we will create vectors of these words, as two different vectors will be created for the same word which we don’t want to. Then, we will convert the string to lowercase as, the word “Good” is different from the word “good”. Now, let’s get our hands dirty by implementing Sentiment Analysis using NLP, which will predict the sentiment of a given statement.
Sentiments have become a significant value input in the world of data analytics. Therefore, NLP for sentiment analysis focuses on emotions, helping companies understand their customers better to improve their experience. We can view a sample of the contents of the dataset using the “sample” method of pandas, and check the no. of records and features using the “shape” method. This is why we need a process that makes the computers understand the Natural Language as we humans do, and this is what we call Natural Language Processing(NLP). And, as we know Sentiment Analysis is a sub-field of NLP and with the help of machine learning techniques, it tries to identify and extract the insights. Add the following code to convert the tweets from a list of cleaned tokens to dictionaries with keys as the tokens and True as values.
AI-based sentiment analysis systems are collected to increase the procedure by taking vast amounts of this data and classifying each update based on relevancy. The potential applications of sentiment analysis are vast and continue to grow with advancements in AI and machine learning technologies. Now that you’ve tested both positive and negative sentiments, update the variable to test a more complex sentiment like sarcasm. In this step, you converted the cleaned tokens to a dictionary form, randomly shuffled the dataset, and split it into training and testing data.
Here’s a detailed guide on various considerations that one must take care of while performing sentiment analysis. A large amount of data that is generated today is unstructured, which requires processing to generate insights. Some examples of unstructured data are news articles, posts on social media, and search history. The process of analyzing natural language and making sense out of it falls under the field of Natural Language Processing (NLP).
‘ngram_range’ is a parameter, which we use to give importance to the combination of words, such as, “social media” has a different meaning than “social” and “media” separately. Now, we will convert the text data into vectors, by fitting and transforming the corpus that we have created. Change the different forms of a word into a single item called a lemma. WordNetLemmatizer – used to convert different forms of words into a single item but still keeping the context intact. The first review is definitely a positive one and it signifies that the customer was really happy with the sandwich.
In some cases, such as datasets with one sentence per line or twitter data, you want to guarantee that there is one sentence per document processed. In the next article I’ll be showing how to perform topic modeling with Scikit-Learn, which is an unsupervised technique to analyze large volumes of text data by clustering the documents into groups. In the script above, we start by removing all the special characters from the tweets. The regular expression re.sub(r’\W’, ‘ ‘, str(features[sentence])) does that.
From time to time, we would like to contact you about our products and services, as well as other content that may be of interest to you. By ticking on the box, you have deemed to have given your consent to us contacting you either by electronic mail or otherwise, for this purpose. NLP-enabled sentiment analysis can produce various benefits in the compliance-tracking region.
For different items with common features, a user may give different sentiments. Also, a feature of the same item may receive different sentiments from different users. Users’ sentiments on the features can be regarded as a multi-dimensional rating score, reflecting their preference on the items. In this article, we saw how different Python libraries contribute to performing sentiment analysis.
Note that the index of the column will be 10 since pandas columns follow zero-based indexing scheme where the first column is called 0th column. Our label set will consist of the sentiment of the tweet that we have to predict. To create a feature and a label set, we can use the iloc method off the pandas data frame. In addition to these two methods, you can use frequency distributions to query particular words. You can also use them as iterators to perform some custom analysis on word properties.
About Jason – Jason Seib adalah pencipta AltShift Diet yang populer, penulis The Paleo Coach, co-host dari JASSA Podcast yang populer, pendiri dan salah satu pemilik AltShift Fitness and Fat Loss, gym kecil yang sukses di area Portland, Oregon, sebagai serta pendiri dan pemilik bersama JassaFIT.com, situs dan komunitas pelatihan online.
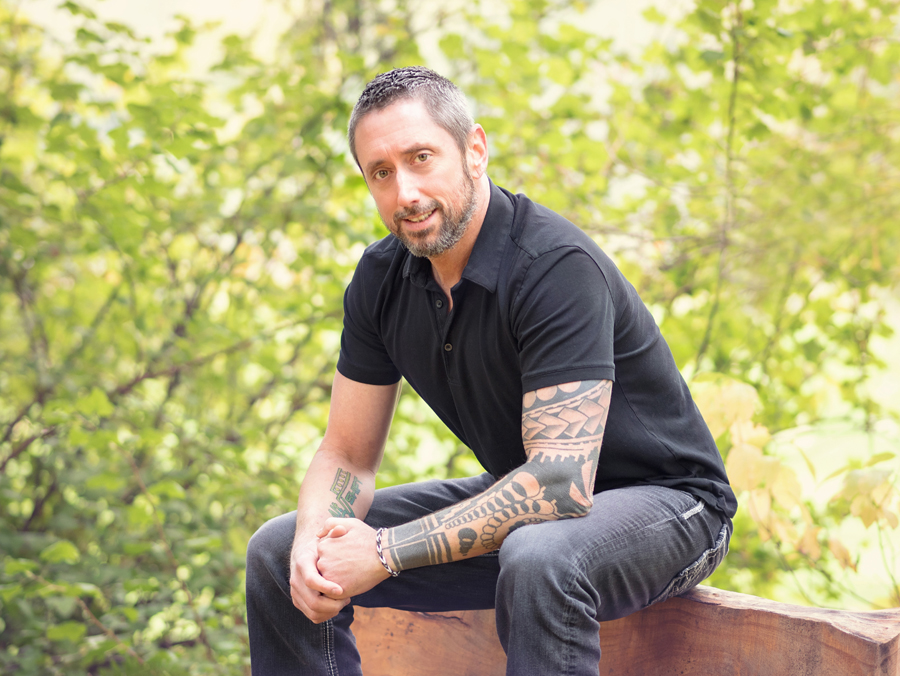
Dia berbicara tentang masalah kehilangan lemak secara teratur, menyampaikan Seminar Penurunan Lemak Berkelanjutan di seluruh AS. Semangat Jason membimbing orang normal ke tingkat kesehatan dan kebugaran yang luar biasa. Menggabungkan pengetahuannya yang luas tentang kebugaran dan nutrisi dengan kecintaannya pada psikologi, ia telah membangun karirnya dengan membantu ribuan orang mengubah perspektif mereka dan menemukan jalan yang sehat dan berkelanjutan untuk mencapai tujuan mereka.
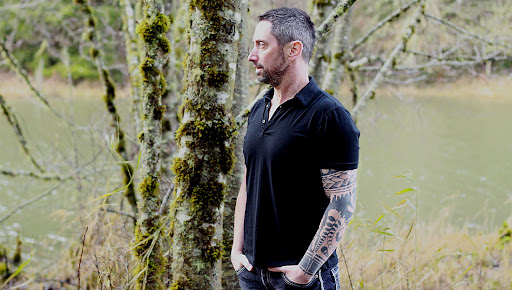
Books by Jason – AltShift – Lasting Fast Loss at Last
AltShift bekerja dengan tidak hanya menanyakan apa yang harus kita makan untuk menjadi sehat dan ramping, tetapi juga kapan kita harus makan makanan tersebut (selama berhari-hari, bukan berjam-jam) untuk memaksimalkan kandungan makronutrien mereka dan untuk menjaga tubuh kita dari beradaptasi dan menghentikan kehilangan lemak.
Bagian yang sulit adalah mempertahankannya? Tidak lagi!
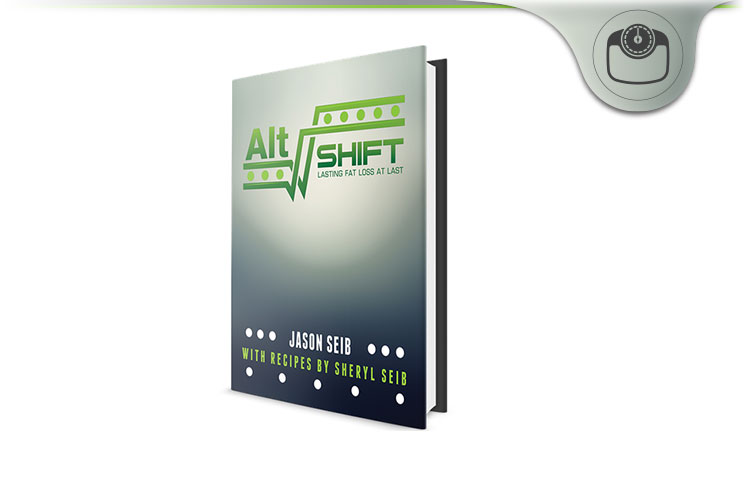
Dapatkan Sekarang
The Fat Loss Directive
Jika Anda ingin sukses jangka panjang, panggilan di semua dasar-dasar gaya hidup Anda akan menjadi penting. Fat Loss Directive menunjukkan jalan dengan 20 tips terpenting kami, menunjukkan kepada Anda di mana harus memfokuskan waktu dan energi Anda yang berharga untuk hasil terbaik. Dapatkan hal-hal ini dengan benar dan sisanya mudah!
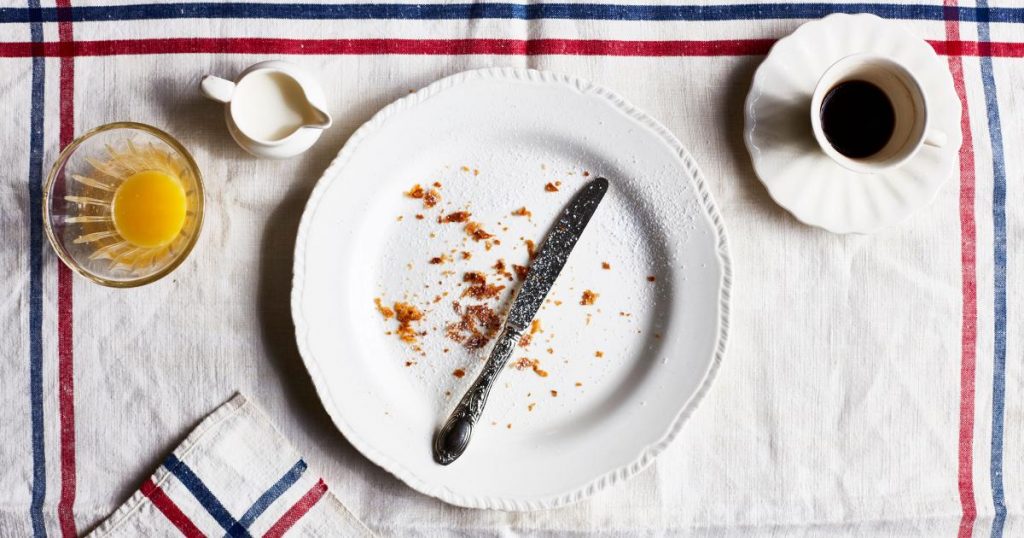
Dapatkan secara gratis
Podcasts – AltShift Podcast, Episode 155 – Pelajaran dari cerita bypass
Omelan saya tentang motivasi, pujian, dan kegilaan yang berasal dari sebuah cerita yang Erica ceritakan kepada saya tentang seseorang di pinggiran dunianya. (9:54) Menurunkan berat badan untuk pernikahan Anda. (44:00) Unduh episode ini AltShift tersedia di sini, AltShift Lift dan AltShift Dish ada di sini.
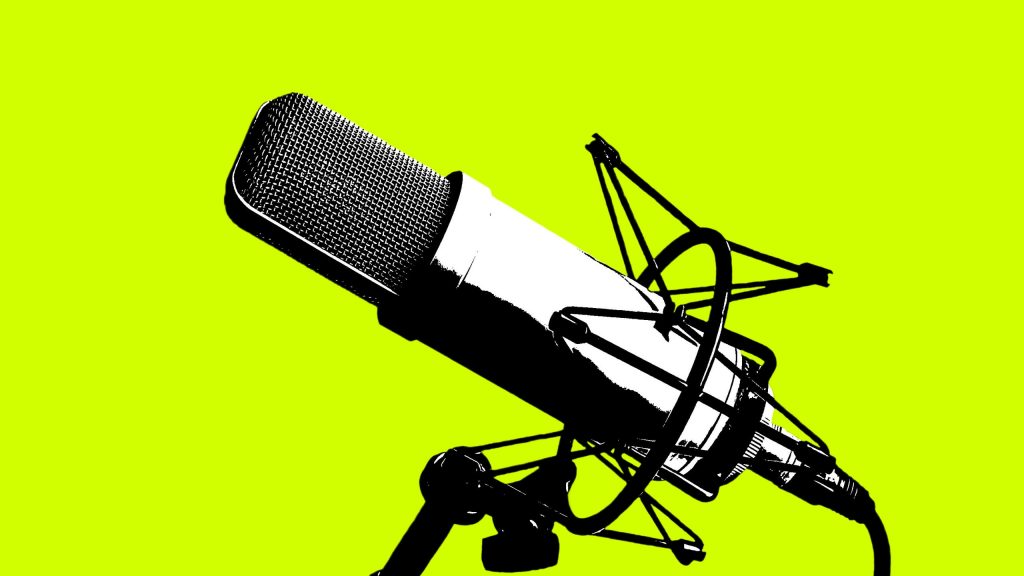
AltShift Podcast, Episode 154 – Mengobati emosi negatif
Mengobati emosi negatif (6:20) Media sosial dan “teman” (22:32) Transformasi Erica (31:33) Download episode ini AltShift tersedia di sini, AltShift Lift dan AltShift Dish ada di sini. Body Beliefs tersedia di Amazon di sini dan sebagai ebook di sini. Forum GRATIS kami ada di sini. Bergabunglah dengan kami di Discord di sini.
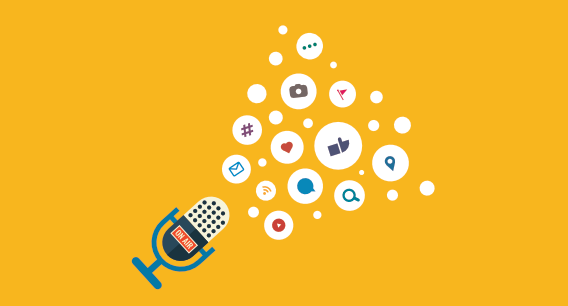
AltShift Podcast, Episode 153 – Jangan ubah dirimu sendiri
Dalam episode ini, kita membahas mengapa mengubah diri sendiri mungkin bukan pendekatan yang tepat. Unduh episode ini AltShift tersedia di sini, AltShift Lift dan AltShift Dish ada di sini, dan AltShift Like A Pro Webinar tersedia di sini. Body Beliefs tersedia di Amazon di sini dan sebagai ebook di sini. Forum GRATIS kami ada di sini.
AltShift Activate – Putaran AltShift Activate Selanjutnya Akan Diumumkan Segera!!
Pantau terus!
Baca apa yang orang katakan tentang bagaimana AltShift Activate mengubah cara berpikir mereka dan berkontribusi pada kesuksesan mereka. Dan kita baru saja mulai!
“Saya memulai Alt-Shift pada hari peluncurannya, dan sementara saya mendapatkan hasil yang baik ketika hidup menjadi stres, saya menghentikan program tersebut. Proses Alt-Shift Activate sangat membantu untuk mempelajari cara menganalisis sisi emosional/mental dari hubungan saya dengan makanan. Wawasan luar biasa seperti itu sangat berharga!” – Cheryl
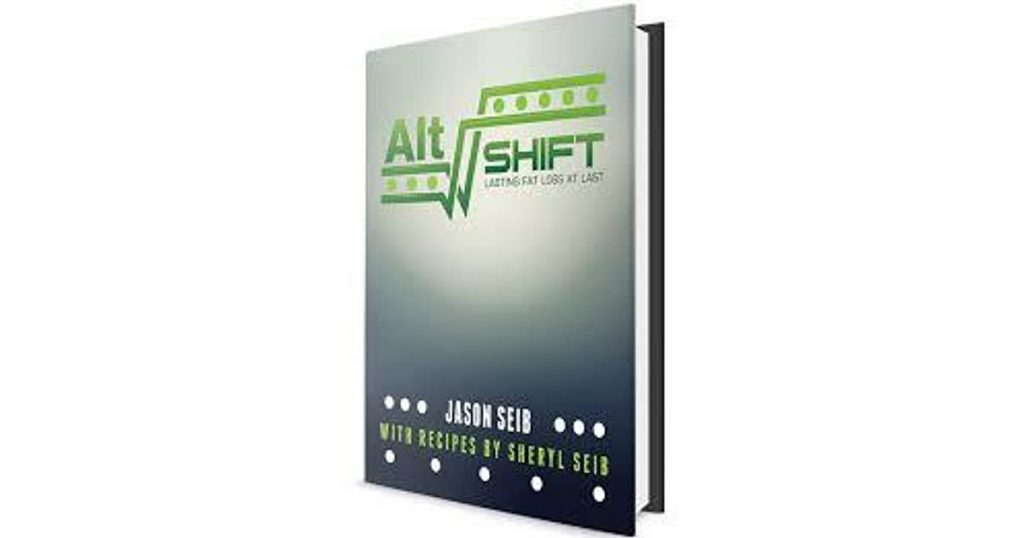
“AltShift Activate jelas membantu saya menyadari pentingnya berjalan, meditasi, dan tidur. Semuanya bekerja sama untuk kesehatan bukan hanya makanan. Video harian sangat memotivasi. Perhatian dan dukungan Jason sangat luar biasa!” – Kimberly
“Saya sudah bisa melepaskan pelacakan setiap gigitan yang saya makan. Sekarang, saya hanya menempatkan makanan di tempatnya di setiap Shift. Saya memiliki kendali sekarang. Saya juga memiliki beberapa momen besar yang membuka mata yang akan sangat membantu untuk memiliki perspektif yang benar tentang tubuh saya. Saya sangat berterima kasih atas video harian Jason. Beberapa sulit didengar, tetapi sangat dibutuhkan! Saya sangat merekomendasikan AltShift Activate kepada siapa pun yang baru atau seseorang yang sudah ada sejak awal seperti saya.” – Mandy
“ASA telah menjadi kunci bagi saya untuk menyatukan semua bagian AltShift. Ini adalah terapi intensif dengan Jason dalam lingkungan kelompok yang mendukung. Saya sangat senang saya mendaftar untuk ASA!” – Jeannine
“AltShift Activate telah menjadi pengubah permainan untuk menempatkan kepala saya mengapa saya menyabotase diri sendiri dan keluar jalur. Kata-kata bijak Jason sangat tepat dan akurat, seolah-olah dia ada di kepala kita!” – Candice
“Saya telah AltShifting sejak awal dan meskipun saya sepenuhnya memahami pedomannya, saya terlalu memikirkannya. AltShift Activate telah menjadi pengubah permainan bagi saya. Mengurai hal-hal seperti yang dilakukan Jason membuat segalanya begitu sederhana dan mudah diikuti. Begitu saya “keluar dari pikiran saya” dan baru mulai mengikuti empat pilar (meditasi, tidur, berjalan, dan makan sesuai dengan giliran kerja saya) rasanya begitu alami dan sesuatu yang bisa saya lakukan selama sisa hidup saya.” – Melissa
“Saya memiliki begitu banyak momen A-HA, sungguh gila! Jason punya cara untuk membuatmu pergi…OH!!! Aku mengerti sekarang! Atau iya!!! Itu AKU! Saya melakukannya! Dia memberi begitu banyak wawasan dan bimbingan, saya selamanya berterima kasih!” – Dre
“Saya berjuang selama 9 bulan dan terus menyimpang setiap beberapa minggu selama beberapa minggu. AltShift Activate telah membantu saya fokus pada pilar yang diperlukan untuk melakukan ini seumur hidup. Bagi saya, dukungan ASA dan Jason sangat berharga.” – Lea
“ASA adalah apa yang Anda butuhkan ketika Anda telah membaca buku, menggunakan AltShift seperti seorang profesional, bergabung dengan grup FB dan Forum dan menyadari bahwa Anda perlu menggali lebih dalam untuk membuat perubahan ini. Menjadi jujur dan rentan pada saat yang sama adalah kunci untuk mengambil bagian dalam Activate. Saya akan mengambil bagian dalam Activate lebih dari sekali karena itu sangat berdampak tetapi saya tidak perlu melakukannya. Saya telah mengubah bagian dalam yang benar-benar mengubah bagian luar saya.” – Jessica
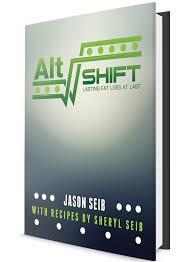
“Di AltShift Activate, saya bangun setiap hari dengan video baru oleh Jason untuk membimbing dan menginspirasi saya saat saya belajar mengintegrasikan AltShift ke dalam kehidupan saya yang sibuk. Video yang informatif namun responsif itu, selain pengingat tugas harian dan komunitas online yang hidup dan menggugah, menawarkan nilai luar biasa dengan biaya minimal. Berinvestasi di AltShift Aktifkan untuk mendapatkan dasar-dasar AltShift dengan cara yang dapat dikelola telah bernilai setiap sen.” – Sally
Body Beliefs Intro – Jenny berusia 6 tahun ketika dia mengetahui bahwa lemak itu buruk.
Dia berpura-pura menjadi kucing, merangkak merangkak dan mengeong saat dia berjalan ke kamar orang tuanya, di mana dia menemukan ibunya berdiri di depan cermin dengan ekspresi jijik di wajahnya dan kedua tangan mencubit lemak di wajahnya. perutnya.
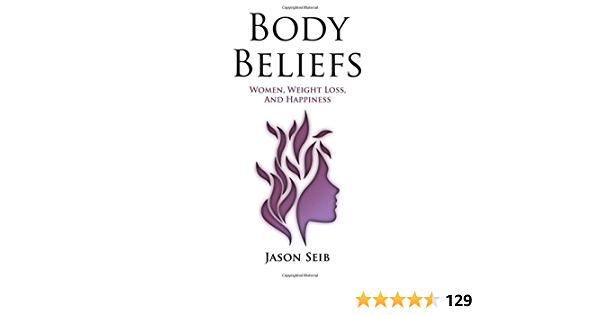
“Apa yang kamu lakukan, Bu?” tanya jennie. “Tidak ada, sayang,” kata ibunya sambil menghela nafas. “Ibu hanya gemuk.”
Jenny bingung. Ibunya adalah wanita tercantik yang dia kenal, tapi ternyata ada yang salah dengan bisa mencubit perutmu.
Jenny mencubit perutnya sendiri dan bertanya-tanya apakah ada yang salah dengannya juga, tetapi dia kebanyakan hanya merasa bangga menjadi seperti ibunya.
Seiring bertambahnya usia Jenny, dia selalu sadar bahwa ibunya tidak bahagia dengan dirinya sendiri.
Pelajaran yang diambil Jenny dari citra tubuh ibunya yang buruk adalah bahwa wanita harus selalu khawatir tentang penampilan mereka, dan terutama tentang angka yang mereka lihat di timbangan kamar mandi mereka.
Jenny kadang-kadang akan berdiri di atas skala ibunya, tetapi dia tidak memiliki kerangka acuan atau alasan apa pun untuk merasa buruk tentang dirinya sendiri, jadi dia tidak menaruh banyak perhatian pada apa yang dia lakukan.
Dia hanya ingin menjadi gadis besar, dan gadis-gadis besar menimbang diri mereka sendiri dan banyak berbicara tentang berat badan mereka.
Kemudian itu terjadi. Suatu hari ketika Jenny berusia 11 tahun, dia mengenakan gaun hitam-putih baru ke sekolah.
Dia merasa sangat bangga dengan penampilannya, dan dia berseri-seri ketika salah satu temannya memperhatikan gaunnya dan memberinya pujian.
Melihat pertukaran itu, seorang anak laki-laki bernama Joseph melihat kesempatan, seperti yang sering dilakukan anak-anak, untuk mendapatkan perhatian dengan menusuk teman sekelasnya.
“Kamu benar-benar menyukai gaun Jenny ?!” dia berteriak. “Dia terlihat seperti sapi perah yang gemuk!”
Jenny merasa malu ketika Joseph lari sambil tertawa bersama dua anak laki-laki lainnya.
Puluhan tahun kemudian, dia masih akan mengingat setiap detail saat itu, hingga aroma rumput segar di taman bermain.
Dua tahun kemudian, Jenny berusia 13 tahun dan imut seperti kancing, tapi dia bukan gadis kecil.
Faktanya, dia adalah “terbesar” dari dua sahabatnya, tetapi itu hanya karena mereka sangat kecil.
Ketika pubertas tiba, itu membawa serta minat pada anak laki-laki dan kesadaran akut akan tubuh di sekitarnya.
Sayangnya, ibu Jenny telah mengajarinya bahwa ukuran sangat penting.
Jenny membandingkan tubuhnya dengan teman-temannya setiap hari dan hampir selalu merasa lebih buruk setiap kali.
Ayah Jenny adalah pria baik yang sangat mencintai ibunya, tetapi pendapatnya sepertinya tidak pernah menjadi masalah dalam hal citra tubuh ibunya.
Dia telah dikalahkan oleh kesadaran yang lambat bahwa, terlepas dari seberapa banyak dia menjilatnya, istrinya akan selalu membenci tubuhnya.
Pada saat Jenny cukup besar untuk memperhatikan hal-hal seperti itu, dia tidak dapat mencoba lagi dan tidak lagi memberikan contoh yang bagus tentang ketertarikan pria.
Lebih buruk lagi, dia adalah laki-laki biasa dan, meskipun dia tidak pernah bermaksud jahat, dia tidak selalu sensitif seperti anak perempuan yang terkadang membutuhkan ayah.
Komentar seperti “Mungkin kamu tidak boleh makan terlalu banyak, sayang” akan melekat di benak Jenny selamanya.
Melompat ke tahun seniornya di sekolah menengah, Jenny membawa apa yang dia perkirakan menjadi tambahan 15 pon lemak.
Itu sebenarnya lebih seperti 8 pound ekstra, tetapi dia sangat ingin menjadi salah satu dari “gadis kurus.”
Dia belum punya pacar yang serius, dan dia yakin ini karena dia gemuk.
Di tahun kedua kuliahnya, Jenny lengah dan berkencan dengan seorang bajingan bernama Jeff.
Ketika dia menangkap Jeff selingkuh, dia melakukan apa yang dilakukan kebanyakan bajingan dan mencoba menyakitinya dalam perjalanan keluar dari pintu dalam upaya untuk membenarkan perilakunya dan meyakinkan dirinya sendiri bahwa dia tidak baru saja kehilangan seorang gadis yang luar biasa.
“Kamu gemuk dan aku selalu tahu aku bisa melakukan yang lebih baik darimu!” Dia berteriak cukup keras untuk didengar tetangga Jenny.
Dia menangis selama dua minggu sementara dia membuat dirinya kelaparan sehingga dia bisa “membuatnya memakan kata-kata itu!”
Setelah beberapa pilihan buruk lagi, Jenny akhirnya menemukan dirinya menikah dengan seorang pria luar biasa bernama Scott yang secara teratur mengatakan kepadanya bahwa dia adalah wanita paling cantik di dunia.
Setiap kali dia mengatakannya, Jenny akan berpikir, “Dia terlalu baik untuk mengatakan bahwa saya gemuk, tapi saya yakin dia berpikir begitu.”
Ketidakamanannya menguras suaminya dengan cara yang sama seperti rasa tidak aman ibunya menguras ayahnya.
Scott akhirnya menjadi frustrasi dengan dia dan cara santai dia mengabaikan pendapatnya, tetapi Jenny salah mengartikan frustrasinya sebagai ketidakpuasan dengan tubuhnya dan bukti bahwa dia benar selama ini.
Dengan setiap upaya penurunan berat badan yang gagal, kerugian sementara akan dibatalkan dengan sedikit lebih banyak berat badan yang diperoleh kembali daripada apa yang telah hilang di tempat pertama.
Setiap “kegagalan” akan merusak jiwanya dan sedikit mengurangi harga diri dan kepercayaan dirinya.
Ketika dia melihat sekeliling dunianya sekarang, Jenny melihat segunung bukti dengan jelas menunjukkan bahwa dia akhirnya akan bahagia jika dia hanya bisa membuat tubuhnya terlihat bagus.
Dari sudut pandang Jenny, semua orang jelas tidak menyukai penampilannya, dan orang-orang tampan jelas lebih bahagia daripada dirinya.
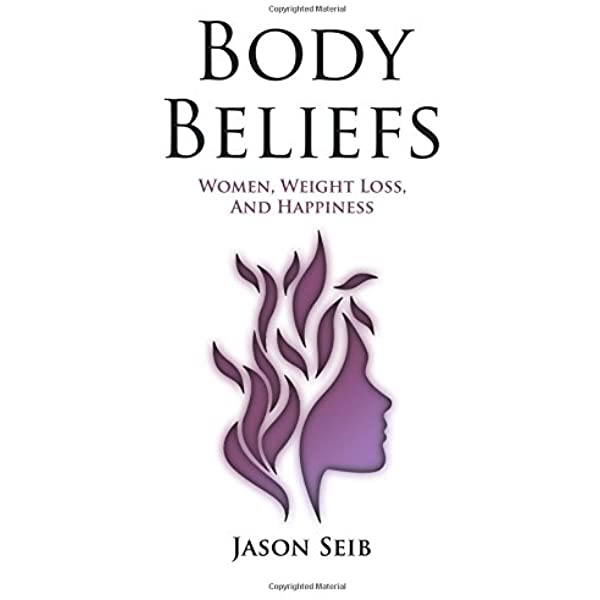
Dengan demikian mengungkap kehidupan yang terlalu umum dari pelaku diet abadi.
Tubuh Jenny adalah musuhnya. Dia membencinya karena penampilannya.
Dia membencinya karena tidak melakukan apa yang dia inginkan setiap kali dia mencoba trik penurunan berat badan yang baru.
Dia membencinya karena menjadi alasan dia tidak pernah benar-benar bahagia.
Dan dia membencinya karena terus-menerus memenuhi pikirannya. Apa yang telah dia lakukan untuk mendapatkan ini?
Sign Up – Menjadi Member Dalam AltShift
Daftar di bawah ini untuk menerima email informatif dan promosi sesekali, serta salinan Body Beliefs GRATIS.
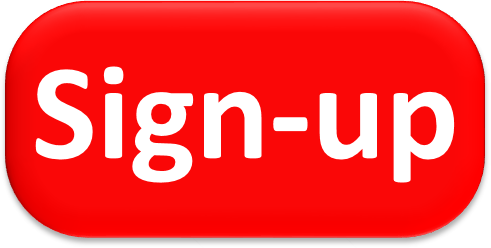
Masukkan nama depan
Masukkan email Anda
Dan jangan khawatir, kami juga membenci spam! Anda dapat berhenti berlangganan kapan saja.
Tetap dalam lingkaran! – Daftar untuk menerima email informatif dan promosi sesekali, serta salinan Body Beliefs GRATIS.
Masukkan nama depan
Masukkan email Anda
Daftar sekarang
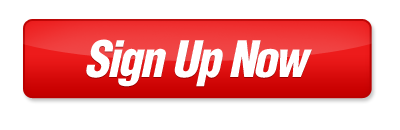
Tetap dalam lingkaran!
Daftar di bawah ini untuk menerima email informatif dan promosi sesekali, serta salinan Body Beliefs GRATIS.
Masukkan nama depan
Masukkan email Anda
Dan jangan khawatir, kami juga membenci spam! Anda dapat berhenti berlangganan kapan saja.
Penutup 3D Body Beliefs -400h
Tips dan Cara Diet Sehat Saat Menginjak Usia 50 Tahun – Menginjak usia pada 50 tahun merupakan tonggak sejarah bagi banyak orang.
Tanda setengah abad hadir dengan aturan baru untuk tes medis dan sering kali membawa beberapa sinyal terkait kesehatan yang menunjukkan bahwa sudah waktunya untuk beberapa perubahan pola makan.
Bahkan bila Anda telah menikmati 50 tahun yang sehat atau lebih, kebutuhan nutrisi berubah dari waktu ke waktu.
Perubahan pola makan bertahap mungkin bijaksana untuk memastikan tahun emas Anda, yah, emas.
Untuk membantu menentukan nutrisi apa yang dibutuhkan tubuh Anda seiring bertambahnya usia, akan sangat membantu jika Anda menjadwalkan konsultasi dengan ahli diet terdaftar.
Pertama, perlu kita ingat bahwa diet bersifat individual. Rekomendasi berubah berdasarkan usia seseorang, tingkat aktivitas, dan masalah kesehatan yang unik.
Akan tetapi, ada beberapa tips yang cocok untuk sebagian besar individu yang lebih tua.
Petunjuk berikut ini dapat membantu pengasuh dan manula yang ingin proaktif mengenai kesehatan dan kualitas hidup mereka.
1. Lindungi Tulang Anda
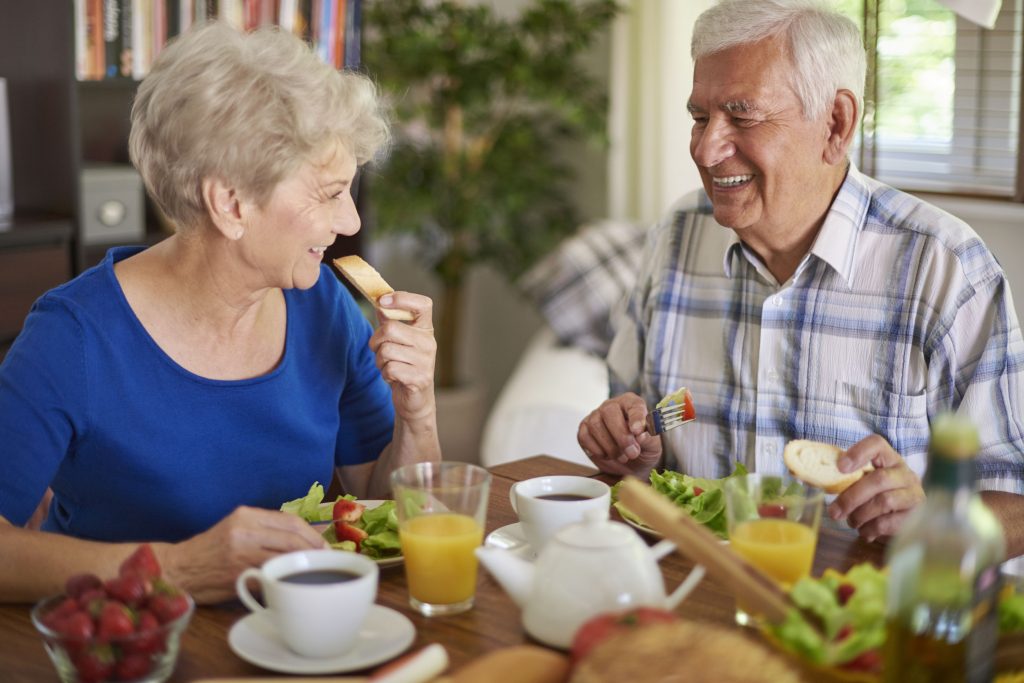
Seiring bertambahnya usia, tulang kita melemah karena penurunan mobilitas dan kehilangan mineral.
Meningkatkan asupan vitamin D dan kalsium hingga tiga kali per hari sangat tepat untuk mencegah osteoporosis atau menjaga kondisi agar tidak semakin parah.
Banyak makanan, seperti sereal, roti dan jus, diperkaya dengan kedua komponen makanan penting ini untuk meningkatkan kesehatan tulang.
National Osteoporosis Foundation juga merekomendasikan untuk meningkatkan kandungan kalsium pada resep dengan menambahkan dua hingga empat sendok makan susu bubuk tanpa lemak.
Setiap sendok makan mengandung 50 mg kalsium, yang dapat membantu Anda mencapai total rekomendasi harian Anda.
Jika Anda merasa Anda atau orang yang Anda cintai tidak mendapatkan cukup mineral dan nutrisi dari makanan yang Anda makan, temui ahli diet terdaftar, praktisi perawat atau dokter untuk mendapatkan resep multivitamin.
Tubuh kita membutuhkan vitamin D yang cukup untuk menyerap kalsium.
Makanan adalah cara terbaik untuk mendapatkan nutrisi, tetapi bisa sulit untuk mencapai asupan yang cukup melalui makanan saja.
Jika gejala tulang melemah atau osteoporosis sudah ada, maka multivitamin dengan tambahan vitamin D adalah pilihan yang lebih tepat.
2. Tingkatkan Tingkat Energi
Ketika kita mencapai usia lima puluhan, adalah umum untuk melihat perubahan tingkat energi harian.
Ini normal sampai batas tertentu, tetapi kekurangan vitamin B12 juga bisa menjadi penyebabnya.
Jika seseorang dites sebagai kekurangan B12, suplementasi harian adalah kuncinya.
Merasa lesu jelas tidak ideal, tetapi menerima penurunan energi dapat menyebabkan penurunan mobilitas dan aktivitas, yang berkontribusi pada osteoporosis, melemahnya jantung, dan perubahan dalam buang air besar.
Kesehatan secara keseluruhan adalah satu lingkaran besar yang saling berhubungan, jadi penting untuk melakukan apa yang diperlukan untuk tetap aktif dan mempertahankan kualitas hidup yang tinggi.
Sumber makanan B12 termasuk hati sapi, mackerel, sarden, daging merah, yogurt dan sereal yang diperkaya.
3. Faktor Dalam Serat
Penyakit yang umum ditemukan pada orang berusia 50 tahun ke atas adalah diabetes tipe 2.
Serat makanan bermanfaat untuk memperlambat pelepasan gula ke dalam aliran darah, yang menurunkan dan menstabilkan kadar glukosa darah.
Serat juga penting untuk pencernaan, menurunkan kolesterol dan membantu menjaga berat badan yang sehat.
Ini akan membantu mempromosikan gerakan usus yang teratur juga.
Pedoman Diet 2015-2020 untuk Orang Amerika merekomendasikan bahwa pria berusia 51 tahun ke atas mengonsumsi 28 gram serat makanan setiap hari dan wanita berusia 51 tahun ke atas harus mengonsumsi 22,4 gram.
Makanan nabati (kacang-kacangan, sayuran, buah-buahan, kacang-kacangan dan biji-bijian) adalah sumber serat terbaik dan cenderung padat nutrisi juga.
4. Ganti Garam
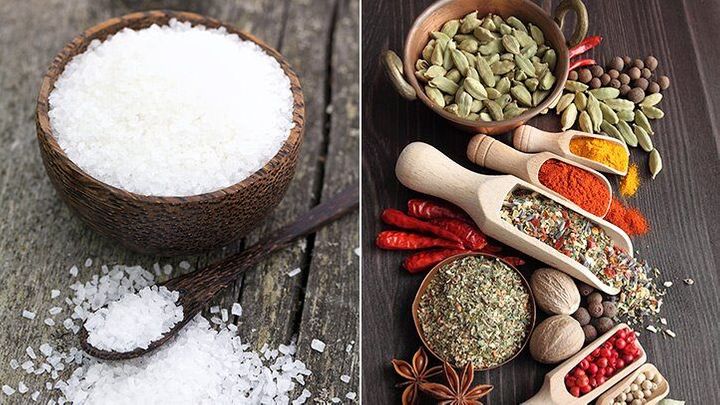
Tekanan darah tinggi bisa menjadi masalah di sekitar usia 50 tahun.
Menghilangkan garam meja adalah salah satu langkah menuju diet jantung sehat.
Cobalah bumbu seperti bubuk bawang putih, bubuk bawang merah, dill, paprika, merica, jeruk, dan rempah segar.
Ada banyak alternatif rendah natrium dan bebas natrium yang bisa Anda masak dengan menambahkan banyak rasa dan sedikit atau tanpa garam pada makanan.
Waspadai kandungan natrium dari saus favorit, bumbu, dan juga makanan yang dikemas dan disiapkan.
Cara termudah untuk memantau asupan natrium adalah dengan menyiapkan makanan rumahan menggunakan bahan-bahan segar.
5. Perhatikan Berat Badan Anda
Hampir setiap senior bertanya-tanya berapa banyak yang harus mereka makan untuk mempertahankan berat badan yang sehat.
Sebagian besar khawatir bahwa mereka mungkin mendapatkan beberapa kilogram saat pulih dari operasi atau kemunduran kesehatan.
Recommended Dietary Allowance (RDA) umum tercantum di bawah ini untuk pria dan wanita senior dengan tingkat aktivitas yang berbeda.
Namun, perlu diingat bahwa ini hanyalah gambaran umum.
Bahkan asupan kalori harus disesuaikan untuk beberapa individu, tergantung pada apakah mereka perlu mempertahankan berat badan yang sehat atau menurunkan/menambah beberapa kilogram.
6. Kesehatan Usus = Kesehatan yang Baik
Prebiotik dan probiotik kadang-kadang disebut “penguat nutrisi.” Prebiotik adalah komponen makanan alami yang tidak dapat dicerna yang meningkatkan fungsi gastrointestinal (GI) dan penyerapan kalsium (yang sangat bagus untuk mereka yang menderita keropos tulang).
Asupan prebiotik dapat ditingkatkan dengan makan hal-hal seperti asparagus, bawang putih, pisang dan makanan gandum.
Probiotik adalah bakteri baik yang secara alami ditemukan di usus.
Antibiotik biasanya diresepkan untuk mengobati infeksi saluran kemih (ISK) dan infeksi lainnya, tetapi obat ini membunuh bakteri baik dan jahat dan dapat berdampak negatif pada sistem GI.
Untuk menjaga flora usus yang sehat dan membantu sistem pencernaan pulih lebih cepat setelah minum obat ini, minum suplemen probiotik setiap hari dan makan makanan seperti yogurt, kefir, asinan kubis, dan kimchi.